Tales from the engineering side of things

Iron is an essential element for producing hemoglobin (the molecule that helps transport oxygen around your body). To promote donor health, we recently made changes to donor eligibility based on new information our researchers have learned about mitigating iron deficiency in blood donors.
When a significant change to donor eligibility occurs, like the recent eligibility changes related to hemoglobin levels and iron health, we need to understand in advance the impact it could have on blood collections.
Our in-house engineer, John Blake, did some nifty number crunching and estimated that the change would mean about 35,000 fewer donations collected in a year — hence the push for new donors to step forward to help fill the gap.
We got to know Dr. Blake a bit better and asked him a few questions about his work calculating the impact of change...
What do you do? What’s your role at Canadian Blood Services?
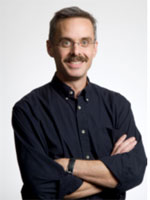
I am an industrial engineer and a professor at Dalhousie University in addition to being a member of Canadian Blood Services’ Centre for Innovation. Besides doing professor-y things like teaching classes and training graduate students, I work on technical challenges that Canadian Blood Services faces in a variety of areas. I’ve worked on inventory and logistics, clinic scheduling, donor flow, stem cell registry composition, and rare blood inventory. Basically, anything that requires a bit of modelling or heavy-duty computing.
As an engineer, I’m a bit different from the rest of my Centre for Innovation colleagues. My scientist friends publish data they collect, like “I cooled platelets to 4oC and I observed that they cleared from the body 40 per cent faster than when held at 22 oC”. Whereas engineers are interested in fitting a model to the data so that we can predict the clearance rate of platelets at any temperature, not just temperature values that we had tested. So, we don’t publish data, we only publish models.
How do you take hypothetical or real-life situations and turn them into math problems? What’s the biggest challenge?
I like working on practical problems, like the ones that pop up in Canadian Blood Services’ operations. Any practical problem is messy — just think of the last time you tried to get your kids (or yourself) ready to go camping for the weekend. People close to a problem usually encapsulate their understanding in a series of “if-then-else” rules. If X happens, then follow process A — unless Y happens, then follow process B. The trickiest part, in my experience, is finding out all the relevant “if-then-else” rules and abstracting from them to get to a model of cause and effect that is both realistic and something that we can actually solve.
How did you go about calculating the impact of this criteria change?
We built a model – an object that helps us predict the behaviour of a real-world element or system in response to changes in inputs. We use models to help explain data that has already been observed and to predict data that has not yet been (or maybe never be) observed.
Estimating the change in donor attendance because of the move to 84-day interval for female donors is a great example of why we build models.
Our job was to come up with a model that represents the behaviour of donors and then as we adjust the inputs in the system, we can observe how the system behaves. So, we built a set of equations that model the rules. This technique is called linear programming. The trick is to find the one solution that gives us the best possible outcome. In the case of iron deferrals, we wanted to find the smallest number of new donors that needed to be added to the donor base to make up for the losses due to the 84-day deferral. Finding the solution to the problem is computationally challenging, but the methods are well known.
The steps:
- We have anonymized data on donors that tells us their usual donation frequency (once per year; twice per year; etc.) and the date of last donation. From this record, we can figure out how many donors are “regular” — meaning those who keep to a consistent frequency — and how many donors are “late” — meaning that they haven’t donated within the past say 26 weeks, if they are a twice per year donor.
- We take the data and try to reconstruct the last year of donation history: How many donors of what variety (late, regular) donated during a particular week? We build this up for both regular and late donors.
- Then we try to predict future donor behaviour after the deferral change has taken place. We do this by estimating that if a donor regularly returns every 56 days and last donated on January 2, that he or she will next donate on Feb 20.
- This brings us to the day of the deferral change:
- At this point, we project regular donors forward according to their deferral period: male 56 days, female 84 days. Actually, for females who donate at mobile clinics, we assume that a more realistic deferral period is 112 days, since clinics tend to rotate back to sites every 56 days, meaning that women will be able to attend only every 2nd mobile clinic in their area.
- We look at the projected blood requirements by hospitals for a particular week and calculate the shortfall in donations.
- At this point we can look in to the future and predict shortfalls in donations. So, we set up a linear programming model that determines the smallest number of people that we need to recruit to make up the shortfall.
- The model can select either a late donor or a new donor. We attach different weights to bringing back a late donor and recruiting a new donor. There is a value to bringing late donors back into the fold — we assume that if we bring them back that they will return to their regular pattern.
- We built a tool for our Canadian Blood Services colleagues in Donor Relations that lets them play with different values for new and returning donors as well as different returning scenarios. Based on those numbers, the team can develop their strategy aimed at recruiting new donors or “late” donors.
We’d never argue that our model was an exact predictor of the future, but it will give some idea of trends and solution scenarios, and helps the organization make evidence-based decisions for donor recruitment
Are there other organizations that calculate predicted impacts change like we do?
I did a bit of a literature search and I didn’t find anyone who had looked at donor schedules in the period following a change in deferrals. However, the donor deferral problem is not unlike the recruiting problems faced by the military: How many privates do they need to recruit this year to ensure that they have enough corporals next year, and enough sergeants in five years’ time, given that x per cent of privates leave per year, and y per cent of corporals leave per year, and so on.
As you can see, Dr. Blake's research hub at Dalhousie University is an important part of our Centre for Innovation. They apply operational research methodologies to address various challenges faced by blood operators. These models provide insight that allows us to make evidence-based decisions to operate Canada’s blood supply chain at the highest possible levels of efficiency, effectiveness, safety and responsiveness.
Canadian Blood Services — Driving world-class innovation
Through discovery, development and applied research, Canadian Blood Services drives world-class innovation in blood transfusion, cellular therapy and transplantation—bringing clarity and insight to an increasingly complex healthcare future. Our dedicated research team and extended network of partners engage in exploratory and applied research to create new knowledge, inform and enhance best practices, contribute to the development of new services and technologies, and build capacity through training and collaboration.
The opinions reflected in this post are those of the author and do not necessarily reflect the opinions of Canadian Blood Services nor do they reflect the views of Health Canada or any other funding agency.
Related blog posts
Led by Dr. Sandra Ramirez, a development scientist at Canadian Blood Services’ Centre for Innovation, this research project led to a new standard that will reduce the number of discarded red blood cell units. By Jenny Ryan and Patrick Walton The issue Since the 1970s, blood operators have limited...
On that front, Canada is doing a very good job. Dr. David Allan, medical director for Canadian Blood Services’ Cord Blood Bank and OneMatch Stem Cell and Marrow Network, reports that more than 1,000 units of cord blood are now available in the public Cord Blood Bank.